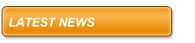 |
|
Search |
|
|
|
|
|
Official Airline |
|
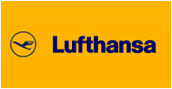 |
|
|
|
|
|
|
|
BEHAVIORAL ANALYSIS AND PERSONALIZATION
Online behavior can range from interaction with services such as Web search engines and recommender systems to the authoring of Web artifacts such as pages, blog posts, and social media updates. As more data are gathered about peoples’ online activities, new techniques are needed to analyze these behaviors and derive actionable knowledge and information. In addition to mined insights, behavioral data can yield a variety of direct user applications, including collaborative filtering and recommendation (that uses the aggregated online behavior of many users), or user modeling and personalization (that may leverage an individual user’s behavior). This calls for novel methods that draw from a wide range of fields such as algorithms, collaborative filtering, data mining, information retrieval, machine learning, and user modeling. It also raises important issues around the preservation of user privacy and the efficiency of algorithms in Web-scale settings.
For the behavioral analysis and personalization track, we invite original and high-quality submissions addressing aspects of the analysis and use of online behavior. The relevant topics include, but are not restricted to, the following:
Topics
- Personalization and recommendation systems
- Query log, click trail, and traffic data mining
- User models, query logs, click logs, personalization
- Collaborative filtering and content ranking using social media
- Other novel Web data mining algorithms
- Efficient algorithms for large-scale analysis
- Spatio-temporal and Uncertain data mining
- Privacy-preserving behavioral analysis
Track Chairs
- Qiaozhu Mei, University of Michigan, USA
- Ryen White, Microsoft Research, USA
Program Committee
- Eytan Adar, University of Michigan, USA
- Eugene Agichtein, Emory University, USA
- Peter Brusilovsky, University of Pittsburgh, USA
- Georg Buscher, DFKI, Germany
- Yunbo Cao, Microsoft Research Asia, China
- Carlos Castillo, Yahoo!, Spain
- Charlie Clarke, Waterloo, Canada
- Deepayan Chakrabarti, Yahoo!, USA
- Raman Chandrasekar, Microsoft Research, USA
- Chen Chen, Google, USA
- Yun Chi, NEC Labs, USA
- Wei Chu, Yahoo!, USA
- Nick Craswell, Microsoft, USA
- Chaabane Djeraba, University of Lille, France
- Doug Downey, Northwestern University, USA
- Georges Dupret, Yahoo!, USA
- Hui Fang, University of Delaware, USA
- Evgeniy Gabrilovich, Yahoo!, USA
- Jaiwei Han, University of Illinois at Urbana-Champaign, USA
- Daqing He, University of Pittsburgh, USA
- Andreas Hotho, University of Würzburg, Germany
- Jeff Huang, University of Washington, USA
- Daxin Jiang, Microsoft Research, China
- Krishnaram Kenthapadi, Microsoft Research, USA
- Dan Kifer, Pennsylvania State University, USA
- Peer Kröger, LMU, Germany
- Srivatsan Laxman, Microsoft Research, India
- Kristen LeFevre, University of Michigan, USA
- Chao Liu, Microsoft Research, USA
- Mark Manasse, Microsoft Research, USA
- Bamshad Mobasher, DePaul University, USA
- G. Craig Murray, University of Maryland, USA
- Rina Panigrahy, Microsoft Research, USA
- Sandeep Pandey, Yahoo!, USA
- Filip Radlinski, Microsoft, UK
- Milad Shokouhi, Microsoft, UK
- Alex Slivkins, Microsoft Research, USA
- Mark Smucker, University of Waterloo, Canada
- Barry Smyth, University College Dublin, Ireland
- Amanda Spink, Loughborough University, UK
- Hanghang Tong, IBM Research, USA
- Xuanhui Wang, Yahoo!, USA
- Ingmar Weber, Yahoo! Research, Spain
- Ji-Rong Wen, Microsoft, USA
- Ran Wolff, University of Haifa, Israel
- Xiaoxin Yin, Microsoft Research, USA
- Kai Yu, NEC Labs, USA
- Yisong Yue, Carnegie Mellon University, USA
- Dell Zhang, University of London, UK
|
|
|
|
|