BIG Track Talks
Towards Fair Human-AI Collaborative Decision Making
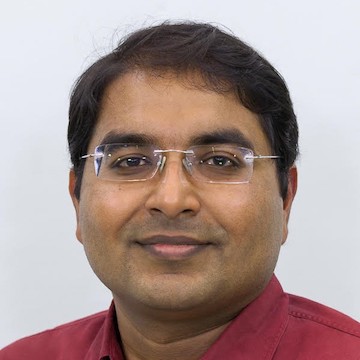
Towards Fair Human-AI Collaborative Decision Making
Krishna Gummadi received the B.Tech. degree from IIT Madras, India, and the Ph.D. degree from the University of Washington, USA. He is currently the Head of the Networked Systems Research Group, Max Planck Institute for Software Systems (MPI-SWS), Saarbrücken, Germany, and a Professor with the University of Saarland, Saarbrücken. Gummadi’s current research interests include understanding and building social computing systems. His current projects focus on enhancing fairness, accountability, transparency, and explainability of automated (particularly, data-driven and learning-based) decision-making systems. Gummadi is also a recipient of numerous awards including the ACM SIGCOMM Test-of-Time Award.
Abstract: Algorithmic (data-driven and learning-based) decision making is increasingly being used to assist or replace human decision making in a variety of domains ranging from banking (rating user credit) and recruiting (ranking applicants) to judiciary (profiling criminals) and journalism (recommending news-stories). Recently concerns have been raised about the potential for bias and unfairness in such algorithmic decisions. Against this background, in this talk, I will present an overview of our recent works tackling the following foundational questions about human-AI decision making:
(a) How do algorithms learn to make biased or unfair decisions?
(b) How can we quantify (measure) and control (mitigate) bias or unfairness in algorithmic decision making?
(c) Can algorithmic decisions be engineered to help humans control (mitigate) bias or unfairness in their own decisions?
Healthy Cities: Tracking Population Health from Grocery Bags and Smart Watches
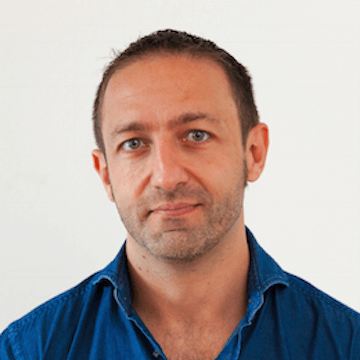
Healthy Cities: Tracking Population Health from Grocery Bags and Smart Watches
Daniele Quercia is Department Head of Social Dynamics at Nokia Bell Labs Cambridge (UK) and Professor of Urban Informatics at the Center for Urban Science and Progress (CUSP) at King's College London. He has been named one of Fortune magazine's 2014 Data All-Stars, and spoke about “happy maps” at TED.
We will see how to aggregate both readings from consumer wearable devices and records of food purchases to track people's well-being at scale. From 11,600 Nokia Health wearables, we collected readings of steps, sleep, and heart rate in the entire cities of London and San Francisco over the course of 1 year. Christmas and New Year’s eve were associated only with short-lived and minor disruptions, while both Brexit and Trump’s election greatly impacted people's sleep and even heart rates. Then, for another entire year in London, we studied the association between food purchases in grocery stores, as measured by the digital traces of customer loyalty cards, and consumption of medicines. Our results show that analytics of digital records of grocery purchases can be used as a cheap and scalable tool for health surveillance: the distribution of the food nutrients is far more predictive of food-related illnesses (e.g., diabetes) than socio-economic conditions.
Reflections of Social Networks
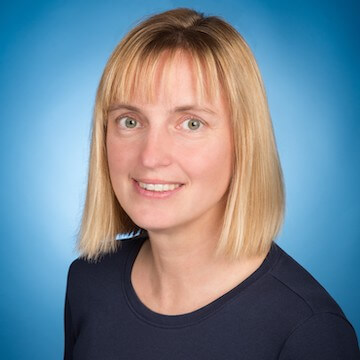
Reflections of Social Networks
Lada Adamic leads the Computational Social Science Team at Facebook. Prior to joining Facebook she was an associate professor at the University of Michigan's School of Information and Center for the Study of Complex Systems. Her research interests center on information dynamics in networks. She has received an NSF CAREER award, a University of Michigan Henry Russell award, the 2012 Lagrange Prize in Complex Systems.
Social networks are shaped by where and how people are brought together as they move through life. Aggregate county-to-county ties in the United States tell of geographical distance but also of characteristics of the counties and past migrations between them. The international movement of migrants shapes international ties and shortens the network distance between any two people in the world. College is a place where new networks form year after year, a process influenced by the type of college and the seasonality of academic life. Finally, coordinated inauthentic behavior on social media leaves its own signature.
Theory and Systems for Weak Supervision
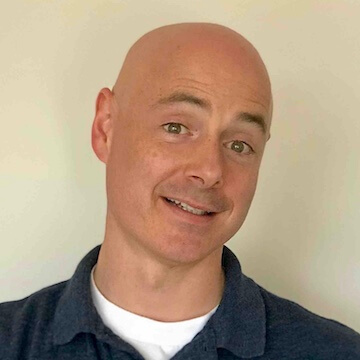
Theory and Systems for Weak Supervision
Christopher Ré is an associate professor affiliated with DAWN, the Statistical Machine Learning Group, and SAIL (bio). Our lab works on the foundations of the next generation of machine-learning systems. While we're very proud of our research ideas and their impact, the lab's real goal is to help amazing students become professors, entrepreneurs, and researchers. With my students and collaborators, I've been fortunate enough to found companies including Lattice, now part of Apple, and SambaNova. The honor that still doesn't feel real is the MacArthur Fellowship.
If you want to build a high-quality machine learning product, build a large, high-quality training set. At first glance, this seems as useful as the statement “if you want to be rich, get a lot of money.” However, a key idea driving our work is that new theoretical and systems concepts including weak supervision, automatic data augmentation policies, and more, can enable engineers to build training sets more quickly and cost effectively.
Along with state-of-the-art results on benchmarks, these concepts have allowed our group and collaborators to build a range of state-of-the-art applications including patient-care monitoring on electronic health records, automatic triage systems for radiologists, and enabling cardiologists to spot rare abnormalities in video MRI—along with widely used products from Apple and Google. This talk describes the theoretical and systems challenges that such applications create.
Much of this work is open source and available at http://snorkel.org.
Big Data with Big Connections
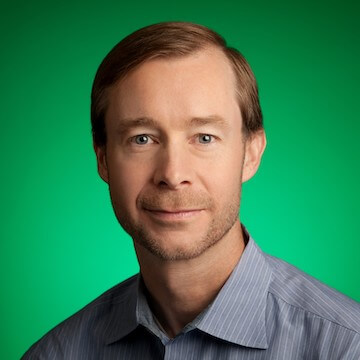
Big Data with Big Connections
Andrew Tomkins received his Ph.D. degree from the Carnegie Mellon University, USA. He is the Director of Google Research. Before Google, he worked at Yahoo! in research and search, and before that he worked at IBM Almaden.
We perform data mining tasks today over huge and growing datasets. To handle scale, we rely on a handful of highly optimized primitive operations.
The current workhorse primitive in training of ML models is stochastic gradient descent, which incorporates per-instance label data efficiently into a model's internal state. However, information is often available to us, not just as labels, but also through connections between data instances, often presented as graphs, sometimes in other forms.
In this talk, Andrew Tomkins will describe a number of different approaches to incorporating such higher-order data into scalable training and inference, and will suggest some open problems in this area.
Food and Health - Sensing, Analytics and Intervention
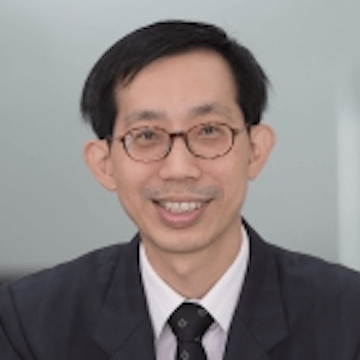
Food and Health - Sensing, Analytics and Intervention
Ee-Peng Lim is the Lee Kong Chian Professor of Information Systems and Director of Living Analytics Research Center (LARC) in the Singapore Management University. He received a PhD in Computer Science from University of Minnesota. His research interests include social network and web mining, information integration, and information retrieval. In LARC, he leads a team of faculty researchers to carry out several big data analytics projects for improving citizens' social wellbeing. Lim has served as the General Chair and Program Chair of several international conferences including PAKDD2020, CIKM2016, ER2019, SocInfo2013, and ASONAM2013.
Eating well is essential to good health. Unfortunately, statistics have shown that most people do not eat well and are thus proned to illnesses such as diabetes and cardiovascular diseases. In the big data era, one can however develop new AI research and technologies to address challenges in collecting food and health data, analyzing data for harnessing food consumption and health insights, as well as developing intervention mechanisms to foster healthy dining behavior. In this talk, we will outline our research on sensing and analysing food and food consumption behavior as well as intervening food choices. We will cover some example research projects in Singapore to illustrate how big data researchers can find opportunities to turn research into real world solutions.
Naver Search: Artificial Intelligence Powered Search Portal
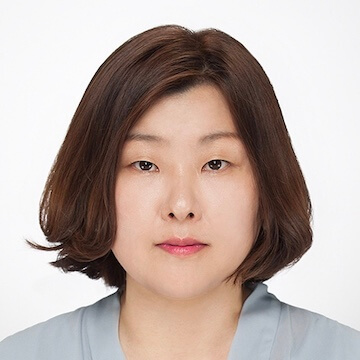
Naver Search: Artificial Intelligence Powered Search Portal
Hyoju Chung is the director of Data Science, Search&Clova at Naver. She receieved a PhD in Biostatistics from University of Washington, USA. Before Naver, she worked at Samsung SDS, Seoul, and Collaborative Health Studies Coordinating Center, Seattle.
Naver is the largest search engine in South Korea. At Naver our mission is to connect people to information that enriches everyday life. Since started in 1999 as a web search portal, Naver has successfully delivered a wide range of information and communication services including Naver Mobile, LINE messenger, SNOW and Webtoon. Continuous innovations in search technology, big data and recommender system play an important role in such success. In this talk we will highlight some ongoing efforts to improve search services, specifically how Naver’s search engine has been powered by Artificial Intelligence (AI). This talk will present recent development and future directions in product-centric AI research of NAVER and LINE.
Advertising Data for Social Good
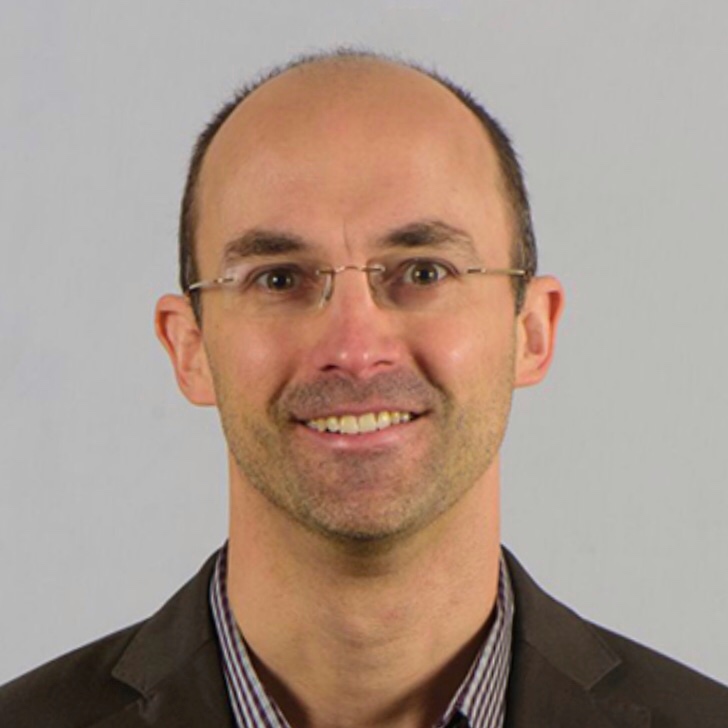
Advertising Data for Social Good
Ingmar Weber is the research director of the Social Computing Group at the Qatar Computing Research Institute (QCRI). His interdisciplinary research looks at what online user-generated data can tell us about the offline world and society at large. He works with sociologists, political scientists, demographers and medical professionals as well as with UN agencies and NGOs in the Data for Development space. Prior to joining QCRI, Dr Weber was a researcher at Yahoo Research Barcelona. As an undergraduate he studied mathematics at the University of Cambridge before pursuing a PhD at the Max-Planck Institute for Computer Science. He is an ACM, IEEE and AAAI Senior Member and serves as an ACM Distinguished Speaker.
Most of the big internet companies, such as Facebook, Google or Twitter, generate their revenue from targeted advertising. To offer advertisers with advanced targeting capabilities, these companies collect large amounts of user data to build elaborate profiles. Based on these profiles an advertiser can then choose to target only, say, female Facebook users living in Taiwan who are aged 35-44, who used to live in the Philippines and who primarily use an iOS device to access Facebook. To help advertisers in planning their advertising campaigns and the related budget needs, the advertising platforms provide so-called audience estimates on how many of their users match the provided targeting criteria. In the example above, Facebook estimates that there are 3700 monthly active matching users (as of January 8, 2020). In this talk I’ll describe how, in close collaboration with different UN agencies, we’re tapping into these audience estimates to (i) monitor international migration, (ii) track digital gender gaps, and (iii) map wealth inequalities. We consistently find that, despite fake profiles and noise in the inference algorithms, data derived from the advertising platforms can provide valuable information that is complementary to other data sources. At the same time, our work shows the risk of identifying vulnerable groups, rather than individuals, which is often not adequately considered in discussions focused on individual privacy.