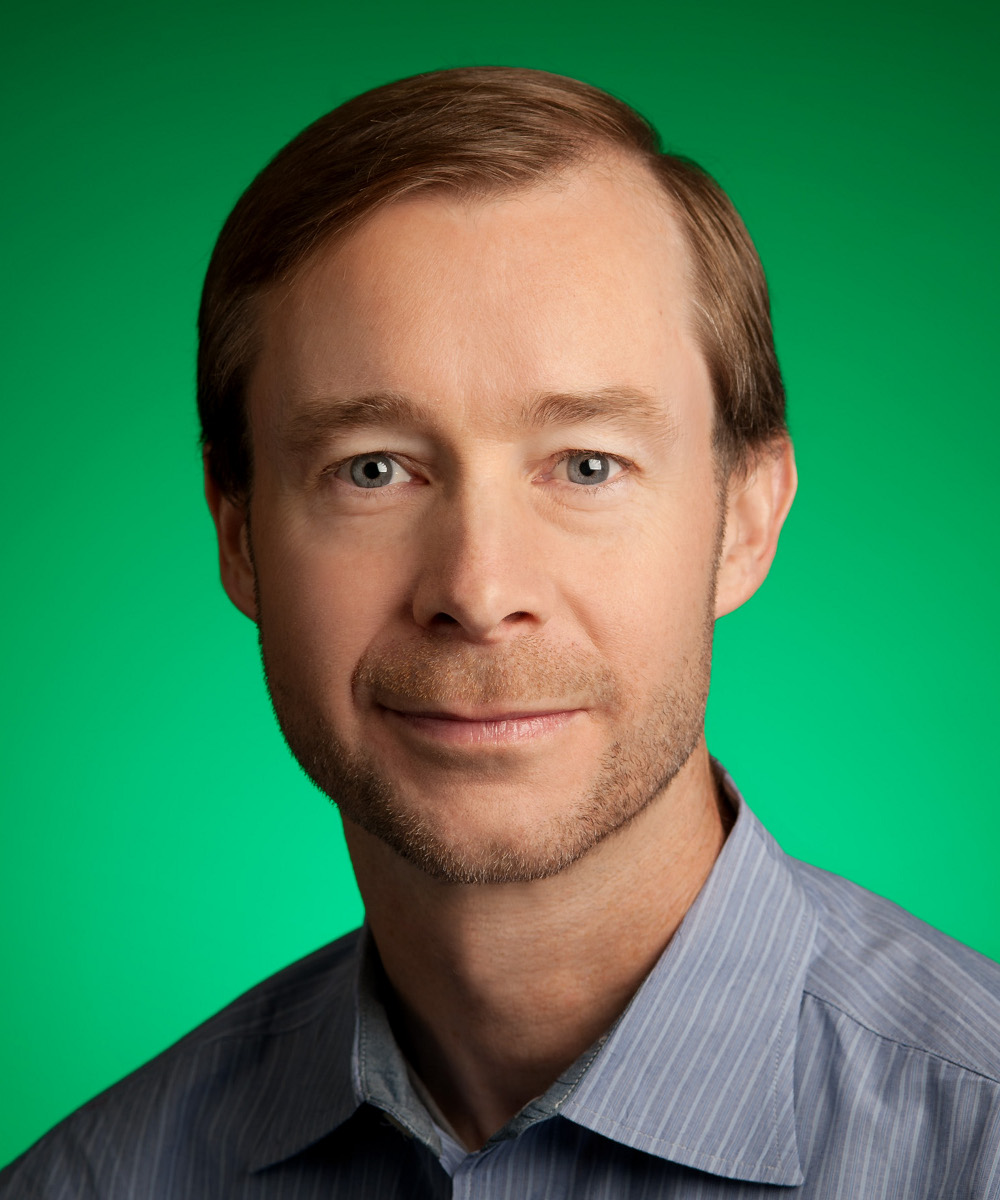
Andrew Tomkins
Andrew joined Google Research in 2009, where he serves as an engineering director working on geo data analysis and machine learning. His earlier research focused on measurement, modeling, and analysis of content, communities, and users on the World Wide Web. Prior to joining Google, he spent four years at Yahoo! serving as chief scientist of search, and eight years at IBM’s Almaden Research Center, where he served as chief scientist on the WebFountain project. Andrew has authored over 100 technical papers and 70 issued patents. He received Bachelors degrees in Mathematics and Computer Science from MIT, and a PhD in CS from Carnegie Mellon University.
Title: When Big Data Meets Discrete Choice
Abstract: In Big Data settings, we often encounter enormous datasets describing events in which a user selects one item from a set of alternatives: one movie to watch, song to play, or restaurant to visit. This is exactly the problem studied in Discrete Choice. Iโll introduce the standard models of choice theory and discuss their accuracy and representative power compared to their scaling properties in modeling large datasets of online behavior. Iโll show some results from example domains ranging from music to product purchase. Finally, Iโll close with some open problems in the domain of user choice modeling.
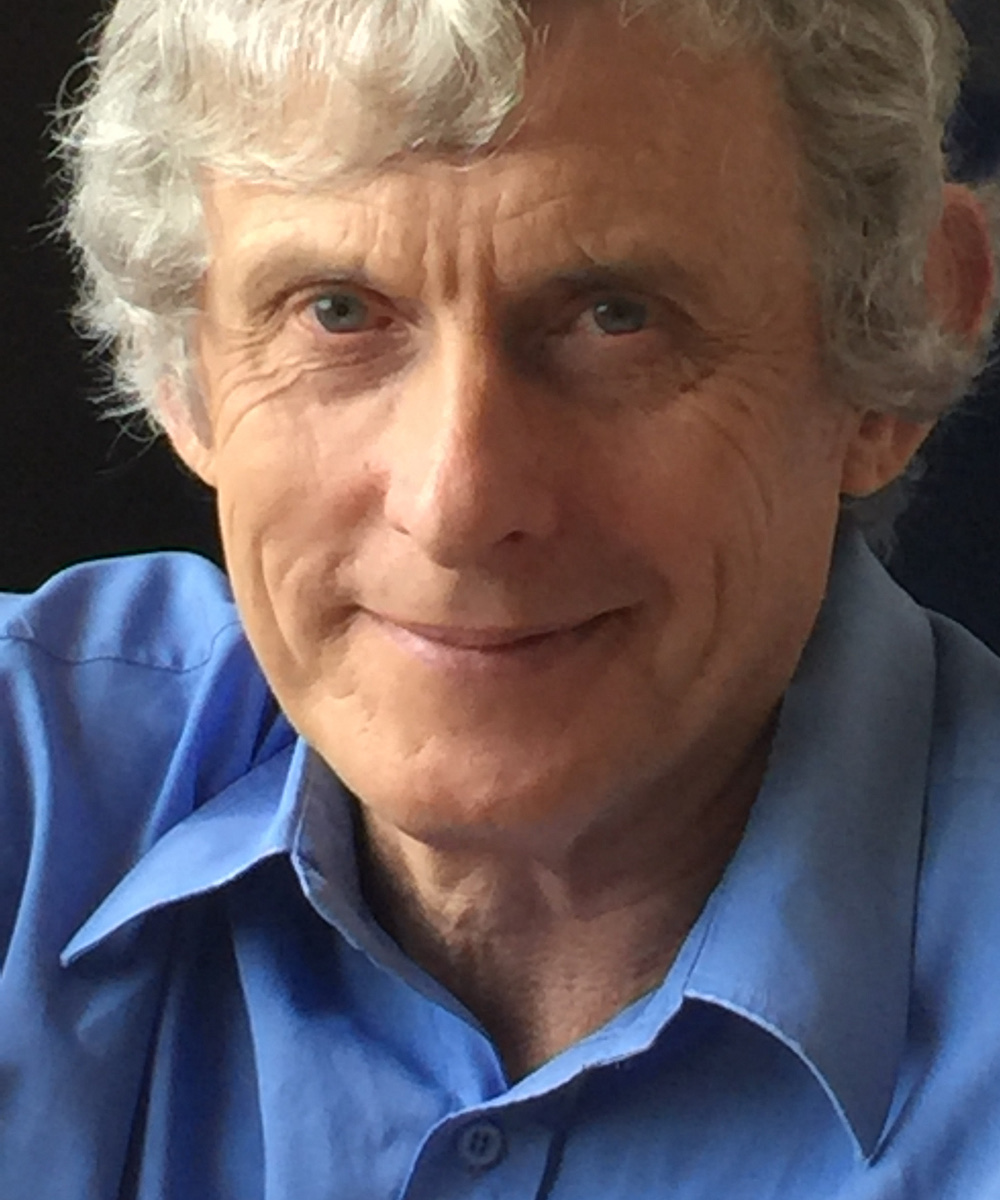
David Hawking
David is a partner architect in the Relevance Sciences and Artificial Intelligence area at Bing (Microsoft) where he has worked on large scale indexing, query autocompletion and low-latency query classification. Prior to joining Microsoft in 2013 he was an IR (Information Retrieval) researcher in academia and government and later technical founder of the Funnelback enterprise and intranet search company.
David and colleagues were responsible for the creation and distribution of a number of “very large” test collections through the TREC Web Track in the 1990s. These were widely used in universities and industry over more than a decade. David’s work in IR has resulted in a number of awards .
Title: Synthesizing large-scale text corpora for training, testing and performance validation of search components.
Abstract: Engineers in cloud-hosting companies are increasingly facing the problem of designing and optimizing search services operating over text datasets to which the engineers have very limited access. Microsoft faces this problem in multi-tenant environments such as Office 365, Exchange 365 and OneDrive. Engineers want to deliver accurate and responsive search over tenant data, but inadvertant leakage of confidential data could cause serious reputational damage. Simulation may be a way of bridging the gap.