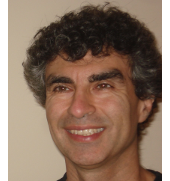
Yoshua Bengio (PhD in CS, McGill University, 1991), post-docs at M.I.T. (Michael Jordan) and AT&T Bell Labs (Yann LeCun), CS professor at Université de Montréal, Canada Research Chair in Statistical Learning Algorithms, CIFAR Fellow, member of NIPS foundation board and former program/general chair.
He co-created ICLR conference, authored 3 books and over 300 publications, the most cited being in the areas of deep learning, which he pioneered, recurrent networks, probabilistic learning, natural language and manifold learning. He is among the most cited Canadian computer scientists and is or has been associate editor of the top journals in machine learning and neural networks.
Abstract
Big Data and Deep Learning for AI
Research in artificial intelligence has known surprising breakthroughs in recent years, thanks in great part to progress in deep learning. So much so that some people now express fears about the potential consequences, whereas just a few years ago the hope for reaching human-level intelligence was gone from most radar screens. Deep learning methods are approaches to machine learning, which allow computers to obtain the knowledge required for intelligent behaviour through learning from examples. More specifically, deep learning algorithms are based on learning multiple levels of representation. Deep learning has already been extremely successful in speech recognition, computer vision and is quickly rising as a major tool for natural language processing. We highlight the theoretical and practical importance of large datasets in these breakthroughs, and over the longer term, in order to approach human-level AI. This begs the question which many researchers currently investigate: how to better exploit the huge unlabeled sets now at our disposal? Research in unsupervised and semi-supervised learning is one of the fundamental challenges for deep learning and we review recent progress.