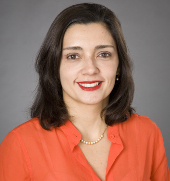
Juliana Freire is a Professor at the Department of Computer Science and Engineering at New York University. She holds an appointment at the Courant Institute for Mathematical Science, is a faculty member at the NYU Center for Urban Science and at the NYU Center of Data Science, where she is also the Director of Graduate Studies.
Her recent research has focused on big-data analysis and visualization, large-scale information integration, provenance management, and computational reproducibility. Prof. Freire is an active member of the database and Web research communities, with over 140 technical papers, several open-source systems, and 10 U.S. patents.
She is an ACM Fellow and a recipient of an NSF CAREER, two IBM Faculty awards, and a Google Faculty Research award. She has chaired or co-chaired several workshops and conferences, and participated as a program committee member in over 70 events.
Her research grants are from the National Science Foundation, DARPA, Department of Energy, National Institutes of Health, Alfred P. Sloan Foundation, Gordon and Betty Moore Foundation, W. M. Keck Foundation, Google, Amazon, the University of Utah, New York University, Microsoft Research, Yahoo! and IBM.
Abstract
Exploring Big Urban Data
Today, 50% of the world’s population lives in cities and the number will grow to 70% by 2050. Cities are the loci of economic activity and the source of innovative solutions to 21st century challenges. At the same time, cities are also the cause of looming sustainability problems in transportation, resource consumption, housing affordability, and inadequate or aging infrastructure. The large volumes of urban data, along with vastly increased computing power open up new opportunities to better understand cities. Encouraging success stories show better operations, more informed planning, improved policies, and a better quality of life for residents. However, analyzing urban data often requires a staggering amount of work, from identifying relevant data sets, cleaning and integrating them, to performing exploratory analyses over complex, spatio-temporal data.
Our long-term goal is to enable interdisciplinary teams to crack the code of cities by freely exploring the vast amounts of data cities generate. This talk describes challenges which have led us to fruitful research on data management, data analysis, and visualization techniques. I will present methods and systems we have developed to increase the level of interactivity, scalability, and usability for spatio-temporal analyses.
This work was supported in part by the National Science Foundation, a Google Faculty Research award, the Moore-Sloan Data Science Environment at NYU, IBM Faculty Awards, NYU School of Engineering and the Center for Urban Science and Progress.